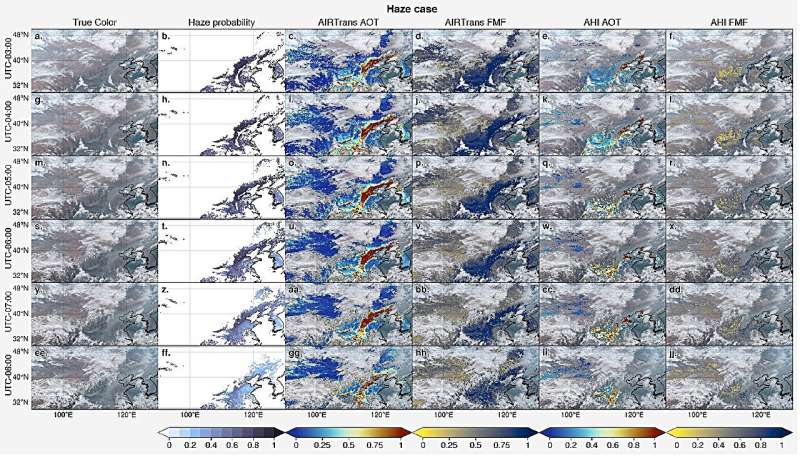
A team led by Prof. Shi Chong from the Aerospace Information Research Institute (AIR) of the Chinese Academy of Sciences, in collaboration with Japanese scientists, achieved a significant milestone in satellite-based air quality monitoring.
They developed AIRTrans, an innovative algorithm that enhances the accuracy and efficiency of retrieving key aerosol properties from multispectral satellite observations. The study is published in the journal Remote Sensing of Environment.
Aerosols, suspended in the atmosphere, are critical for air pollution and climate change. Two important metrics for aerosols are aerosol optical thickness (AOT), measuring particle concentration in the air, and fine-mode fraction (FMF), indicating the proportion of small particles, like those from vehicle emissions or industrial processes.
The model is refined using ground-based measurements to enhance accuracy and adaptability to different real-world situations. Additionally, AIRTrans considers hourly changes in surface reflectance and background AOT, further enhancing precision.
AIRTrans is designed for use with multispectral instruments like the Advanced Himawari Imager (AHI) on Himawari-8, which observes East Asia every 10 minutes. This capability allows for high-frequency tracking of aerosol changes throughout the day, something traditional methods struggle to achieve.
When compared to ground-based measurements, AIRTrans achieved a root mean square error of 0.132 for AOT and 0.146 for FMF, marking significant improvements of about 40% and 49%, respectively, compared to the official Himawari-8/AHI products.
AIRTrans successfully addressed the systematic underestimation of FMF seen in existing retrieval products. During real-world events like haze episodes, AIRTrans effectively captured the dynamics of concentrations and particle sizes, highlighting its effectiveness for pollution monitoring and early warning purposes.
This study demonstrates that AIRTrans is accurate, fast, and widely applicable. It can be adjusted for use with other multispectral satellite sensors, supporting precise air pollution control and related efforts, especially in the context of carbon neutrality goals.
More information:
Chenqian Tang et al, Development of a hybrid algorithm for the simultaneous retrieval of aerosol optical thickness and fine-mode fraction from multispectral satellite observation combining radiative transfer and transfer learning approaches, Remote Sensing of Environment (2025). DOI: 10.1016/j.rse.2025.114619