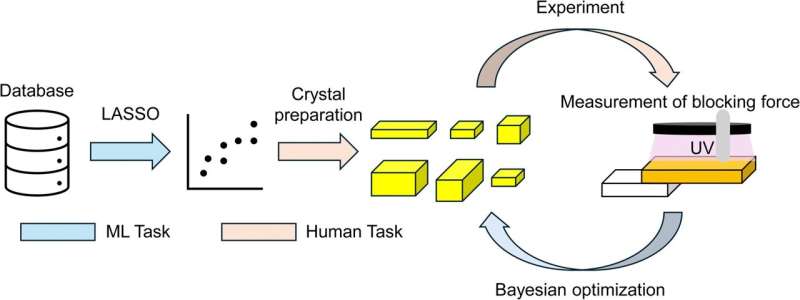
Researchers have created a machine-learning process to enhance the force output of photo-actuated organic crystals. By using LASSO regression to identify crucial molecular substructures and Bayesian optimization for efficient sampling, they achieved a maximum blocking force of 37.0 mN—73 times more efficient than traditional methods.
These results, published in Digital Discovery, could aid in the development of remote-controlled actuators for medical devices and robotics, supporting applications like minimally invasive surgery and precise drug delivery.
Materials that transform into mechanical motion, known as actuators, are essential in robotics, medical devices, and other advanced applications. Photomechanical crystals deform in response to light, making them ideal for lightweight and remotely controlled actuation. Their performance is influenced by factors such as molecular structures, crystal properties, and experimental conditions.
One of the critical performance indicators for these materials is blocking force—the maximum force exerted when deformation is completely restricted. However, achieving high blocking forces is challenging due to the complex interaction of crystal characteristics and testing conditions. Understanding and optimizing these factors are crucial for expanding the potential applications of photomechanical crystals.
In an effort to optimize the force output of photo-actuated organic crystals, researchers from Waseda University have employed innovative techniques to enhance their performance. The study was led by Associate Professor Takuya Taniguchi from the Center for Data Science, along with Mr. Kazuki Ishizaki and Professor Toru Asahi from the Department of Advanced Science and Engineering, Graduate School of Advanced Science and Engineering at Waseda University.
“We observed that machine learning simplifies the search for optimal molecules and experimental parameters,” explains Dr. Taniguchi. “This inspired us to combine these techniques with synthetic chemistry, allowing us to quickly identify new molecular designs and experimental approaches to achieve high-performance outcomes.”
In this study, the team utilized two machine learning techniques: LASSO regression for molecular design and Bayesian optimization for selecting experimental conditions. The initial step led to a material pool of salicylideneamine derivatives, while the latter facilitated efficient sampling from this pool for practical force measurements.
As a result, the team successfully maximized the blocking force, achieving up to 3.7 times greater force output compared to previously reported values, and doing so at least 73 times more efficiently than traditional trial-and-error methods.
“Our research represents a significant advancement in photo-actuated organic crystals by systematically applying machine learning,” notes Dr. Taniguchi. “By optimizing both molecular structures and experimental conditions, we have demonstrated the potential to significantly improve the performance of light-responsive materials.”
The proposed technology has widespread implications for remote-controlled actuators, small-scale robotics, and energy-efficient systems. Because photo-actuated crystals respond to light, they allow for contactless and remote operation, making them suitable for robotic components operating in confined or sensitive environments. Their ability to generate force non-invasively with focused light could also be valuable for microsurgical tools and drug delivery mechanisms requiring precise, remote actuation.
By leveraging a cleaner energy source—light irradiation—while maximizing mechanical output, these materials have the potential for eco-friendly manufacturing processes and devices aimed at reducing overall energy consumption. “Beyond enhancing force output, our approach sets the stage for more sophisticated, miniaturized devices, from wearable technology to aerospace engineering and remote environmental monitoring,” adds Dr. Taniguchi.
In conclusion, this study underscores the power of a machine learning-driven approach in accelerating the development of high-performance photo-actuated materials, bringing them closer to real-world applications and commercial viability.
More information:
Kazuki Ishizaki et al, Machine learning-driven optimization of the output force in photo-actuated organic crystals, Digital Discovery (2025). DOI: 10.1039/D4DD00380B